The Hidden Cost of Single AI Model Thinking
Zero-to-Solve AI Strategy Ai Budget Orchestration Multi-Model AI Strategy Mixture of Experts Enterprise LLM May 12, 2025 7:00:00 AM Steven Muir-McCarey 16 min read
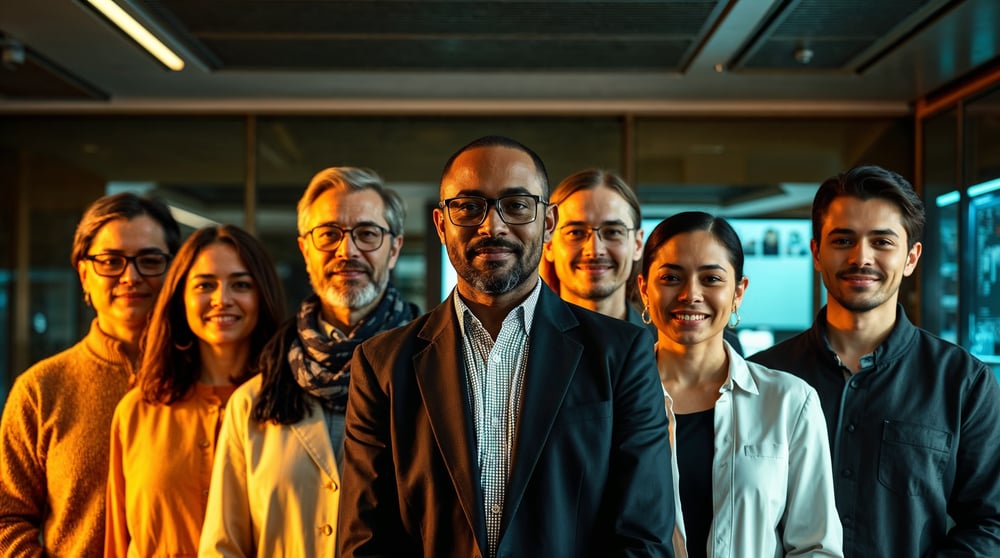
"Is your AI strategy delivering results, or just running up the bill?
OpenAI has announced it will deprecate GPT-4.5 in July. It costs 30 times more than GPT-4o, yet offers only marginal gains. This isn't just a pricing change. It's a signal.
Many organisations are still deploying AI like it's 2022, one large model, stretched across every use case. That approach isn't scalable or cost-efficient. It's a liability.
Today, there's increasing access to fit-for-purpose models from open-source options like Mixtral and LLaMA to enterprise tools like Claude, Gemini and GPT-4o. Each brings different strengths depending on the task, domain or cost to value.
The companies getting this right aren't choosing "the best model." They're orchestrating across many. They route simple prompts to efficient models and reserve premium power for high-value use cases.
This article breaks down the real cost of single-model thinking and what it takes to build a smarter, leaner AI strategy in 2025.
The Era of One-Model AI Is Over
Not long ago, deploying AI meant choosing a model and pushing it across as many use cases as possible. That strategy might have been passable in 2022, but with what we have available now, it's not just an outdated approach, it's an expensive one at that.
We've moved from only having access to a handful of generalist models to many purpose built models and although this could be seen as noise, it's more of a lever waiting to be orchestrated. Even within just the big players we see that OpenAI offers GPT-4o, o4-mini and 5+ other models on their platform. Google provides Gemini 2.5 in both "Pro" and "Flash" editions as well as 10+ other variants. Anthropic's Claude 3.7 Sonnet introduces hybrid reasoning variants and flexible pricing and access to 3+ other variants of its models.
What matters isn't just the name or brand. It's that the cost-performance delta between these models is now massive.
- GPT-4.5: $75 per million input tokens, $150 for output, with only 7% performance improvement over GPT-4o (Ojha, 2025; TechCrunch, 2025)
- GPT-4o: $2.50 input / $10 output per million
- Claude 3.7 Sonnet: $3 input / $15 output, and it handles multi-step reasoning (Anthropic, 2025)
- Gemini 2.5 Pro: $2.50 input / $15 output per million
- Gemini 2.5 Flash: $0.15 input / $0.60 output for fast-response tasks
Use the wrong model for the wrong task and your costs don't double, they can increase by thousands of percent.
Behind the Curtain: Smarter Models, Not Just Bigger Ones
The evolution of AI models in 2025 is marked by a strategic shift towards Mixture of Experts (MoE) architectures, with a focus on efficiency and specialisation over brute-force scale.
Meta's Llama 4 series, released in April 2025, exemplifies this approach. Models like Llama 4 Scout and Maverick use MoE designs that activate only a fraction of their total expert pathways per task. For example, Llama 4 Maverick includes 128 experts, but uses just 17 billion active parameters per inference, a configuration that significantly reduces compute costs while retaining high performance (Dataconomy, 2025).
But perhaps more importantly, enterprises are now beginning to design and fine-tune bespoke MoE models for specific domains: customer service, finance, compliance triage. These tailored models don't aim to outperform general-purpose giants like GPT-4. Instead, they deliver 90% of the value at a fraction of the cost, while aligning tightly with business objectives.
This isn't just a technical upgrade. It's a strategy shift away from "best model overall" toward "best model for this job, at this price."
As model orchestration matures, modularity becomes a competitive lever. Companies embracing smaller, task-specific MoEs are gaining precision, control, and commercial agility.
What Orchestration Looks Like in Practice
Some companies have already made the shift.
A major auto manufacturer uses Microsoft's lightweight Phi model to handle the bulk of incoming customer queries. Only the edge cases get routed to GPT-4o. The orchestration reduced their AI spend by 80 percent, while improving response quality.
Sage, the accounting software company, fine-tuned Mistral for their domain, using it as a triage layer before escalating to a larger model. It improved resolution accuracy and delivered faster responses.
This is what orchestration looks like. It's not just better performance, it's operational leverage.
Vendors are starting to catch up:
- Azure AI Studio now offers over 1,800 models with routing capabilities
- AWS Bedrock provides a unified interface for multiple foundation models
- Google Vertex AI hosts more than 200 models in its Model Garden
Startups like Martian are building routing layers that dynamically switch models based on task complexity. Customers report 70 to 90 percent reductions in model costs with no drop in output quality (TechCrunch, 2025).
Two Strategies, Same Use case, Radically Different Outcomes.
Let's look at how this plays out in practice. Below, two companies deploy models across similar use cases, but only one does it with orchestration in mind.
Feature | Company A: "Premium Everything" Approach | Company B: "Right Tool for the Job" Approach |
---|---|---|
Primary AI Strategy | Use Claude 3.7 Sonnet for all tasks, ensuring premium quality across the board. | Route tasks to the most appropriate model based on complexity and performance requirements. |
Models Used & Task Allocation |
Claude 3.7 Sonnet exclusively for: • Customer Support FAQs (7M interactions) • Investment Research Summaries (2M interactions) • Real-time Trading Analysis (1M interactions) |
Optimized Mix: • Gemini 2.5 Flash for Customer Support FAQs (7M interactions) • GPT-4.1 for Investment Research Summaries (2M interactions) • Claude 3.7 Sonnet for Real-time Trading Analysis (1M interactions) |
Token Calculation (Monthly) |
Input: 10M interactions × 500 tokens = 5,000M tokens Output: 10M interactions × 150 tokens = 1,500M tokens |
Input: (7M × 500) + (2M × 500) + (1M × 500) = 5,000M tokens Output: (7M × 150) + (2M × 150) + (1M × 150) = 1,500M tokens |
Estimated Monthly AI Spend |
Claude 3.7 Sonnet (100% volume):
Input Cost: 5,000M × $3/M = $15,000 Output Cost: 1,500M × $15/M = $22,500 Total: $37,500
|
Gemini 2.5 Flash (70% volume):
Input: 3,500M × $0.15/M = $525 Output: 1,050M × $0.60/M = $630 Subtotal: $1,155 GPT-4.1 (20% volume):
Input: 1,000M × $2/M = $2,000 Output: 300M × $8/M = $2,400 Subtotal: $4,400 Claude 3.7 Sonnet (10% volume):
Input: 500M × $3/M = $1,500 Output: 150M × $15/M = $2,250 Subtotal: $3,750 Total: $9,305
|
Outcomes & Efficiency |
• Customer FAQs: Excellent quality but massive overkill. Slower response times than Flash. 26x higher cost than necessary.
• Research Summaries: Excellent quality, but at premium price.
• Trading Analysis: Excellent quality, appropriately resourced.
• Overall: Consistently high quality but severe cost inefficiencies and suboptimal user experience for high-volume tasks.
|
• Customer FAQs: Perfect quality for use case, 5-10x faster responses, minimal cost.
• Research Summaries: Better document analysis with GPT-4.1's 1M token context and engineering focus.
• Trading Analysis: Top-tier analysis with Claude 3.7 Sonnet for critical decisions.
• Overall: Task-optimized performance, superior user experience, maximum ROI.
|
Business Impact | Very high operational costs. Budget inefficiently allocated to low-complexity tasks. Customer frustration with slower response times for simple queries. Premium pricing may need to be passed to clients. |
75% reduction in AI costs ($28,195 monthly savings)
Faster customer service resolution. Better research quality with specialized model. Demonstrates sophisticated AI strategy. Competitive advantage through efficiency.
|
*Pricing approximate & in USD. Source: OpenRouter.ai
The only difference is that Company B didn't just choose models, they built a model strategy.
What Smart AI Strategy Looks Like
The orchestration approach isn't about jumping on the next new model. It's about building a framework that supports:
- Task audits – Start with what each part of your business needs
- Cost-to-value mapping – Not every prompt deserves frontier-level performance
- Routing logic – Route based on complexity and risk, not convenience
- Ongoing optimisation – Measure performance/cost by model and task type and adjust routing rules accordingly.
This is what separates maturity from experimentation. Without it, you're not building AI systems, you're running expensive experiments at scale.
Time to Unpack What's Really Happening
Companies still relying on single-model AI deployments will be running tech strategies from a previous era. It's no longer enough to have "an AI model." You need a way to manage, optimise, and evolve your AI footprint in line with business goals.
"The winners in this economy won't have the best model. They'll have the clearest orchestration strategy.
Let's Make Sense of It
Curious where your AI investments might be underperforming?
Our Spark Workshop is designed to help leadership teams unpack what's really happening across their AI stack.
We'll help you:
- Identify where model decisions are driving unnecessary costs
- Pinpoint orchestration opportunities already hiding in your business
- Explore how intelligent routing can unlock performance and cost control
This isn't about switching platforms. It's about getting clarity.
Book a Spark WorkshopReferences
Anthropic. (2025). Claude 3.7 Sonnet and Claude Code. https://www.anthropic.com/news/claude-3-7-sonnet
Ars Technica. (2025). "It's a lemon": OpenAI's largest AI model ever arrives to mixed reviews. https://arstechnica.com/ai/2025/02/its-a-lemon-openais-largest-ai-model-ever-arrives-to-mixed-reviews/
Meta / Llama 4:
Dataconomy. (2025, April 7). Meta launches new Llama 4 AI models: Scout, Maverick now available in apps. Retrieved from https://dataconomy.com/2025/04/07/meta-launches-new-llama-4-ai-models-scout-maverick-now-available-in-apps/?utm_source=chatgpt.com
Mistral / Mixtral MoE:
Artetxe, M., Rabe, M. N., Scao, T. L., Tunstall, L., de Masson d'Autume, G., & Elbayad, M. (2024). Mixtral of MoE: Sparse mixture of experts models with open weights (arXiv:2401.04088). arXiv. https://arxiv.org/abs/2401.04088
Google Cloud. (2025). Vertex AI Pricing. https://cloud.google.com/vertex-ai/generative-ai/pricing
Ojha, A. (2025). The great paradox: Why OpenAI's most expensive model GPT-4.5 falls short of expectations. https://medium.com/@ayushojha010/the-great-paradox-why-openais-most-expensive-model-gpt-4-5-falls-short-of-expectations-4c3c5035a692
TechCrunch. (2025). OpenAI plans to phase out GPT-4.5. https://techcrunch.com/2025/04/14/openai-plans-to-wind-down-gpt-4-5-its-largest-ever-ai-model-in-its-api/